20. Xarray Fundamentals#
Attribution: This notebook is a revision of the xarray
Fundamentals notebook by Ryan Abernathy from An Introduction to Earth and Environmental Data Science.
In this lecture, we will have a deep dive to xarray
package. So far in this course, we have used rioxarray
for raster data processing, and stackstac
for analyzing stacks of satellite imagery. Both of these packages are built on top of xarray
; however, we haven’t use all the functionalities of xarray
yet.
In the lecture on Raster Data Processing, we introduced Dataset
in rioxarray
and discussed how it is different from DataArray
. Here we will have a complete review of data structures in xarray
and learn how to create a DataArray
or Dataset
from scratch.
You can access this notebook (in a Docker image) on this GitHub repo.
20.1. Xarray data structures#
Like Pandas, xarray has two fundamental data structures:
a
DataArray
, which holds a single multi-dimensional variable and its coordinatesa
Dataset
, which holds multiple variables that potentially share the same coordinates
20.1.1. DataArray#
A DataArray
has four essential attributes:
values
: anumpy.ndarray
holding the array’s valuesdims
: dimension names for each axis (e.g.,('x', 'y', 'z')
)coords
: a dict-like container of arrays (coordinates) that label each point (e.g., 1-dimensional arrays of numbers, datetime objects or strings)attrs
: anOrderedDict
to hold arbitrary metadata (attributes)
Let’s start by constructing some DataArrays manually
import numpy as np
import xarray as xr
from matplotlib import pyplot as plt
da = xr.DataArray([9, 0, 2, 1, 0])
da
<xarray.DataArray (dim_0: 5)> Size: 40B array([9, 0, 2, 1, 0]) Dimensions without coordinates: dim_0
A simple DataArray without dimensions or coordinates isn’t much use. We can add a dimension name…
da = xr.DataArray([9, 0, 2, 1, 0], dims=['x'])
da
<xarray.DataArray (x: 5)> Size: 40B array([9, 0, 2, 1, 0]) Dimensions without coordinates: x
But things get more interesting when we add a coordinate:
da = xr.DataArray([9, 0, 2, 1, 0],
dims=['x'],
coords={'x': [10, 20, 30, 40, 50]})
da
<xarray.DataArray (x: 5)> Size: 40B array([9, 0, 2, 1, 0]) Coordinates: * x (x) int64 40B 10 20 30 40 50
This coordinate has been used to create an index, which works very similar to a pandas
index.
In fact, under the hood, xarray
uses pandas
index.
da.indexes
Indexes:
x Index([10, 20, 30, 40, 50], dtype='int64', name='x')
xarray
has built-in plotting, like pandas
.
da.plot(marker='o')
[<matplotlib.lines.Line2D at 0xffff58e55950>]
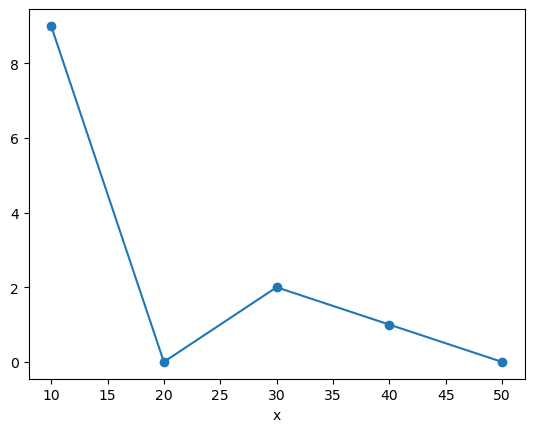
20.1.2. Multidimensional DataArray#
If we are just dealing with 1D data, pandas
and xarray
have very similar capabilities. xarray
’s real potential comes with multidimensional data.
In this example, we use real data from ocean profiling floats. ARGO floats are autonomous robotic instruments that collect Temperature, Salinity, and Pressure data from the ocean. ARGO floats collect one “profile” (a set of measurements at different depths or “levels”).
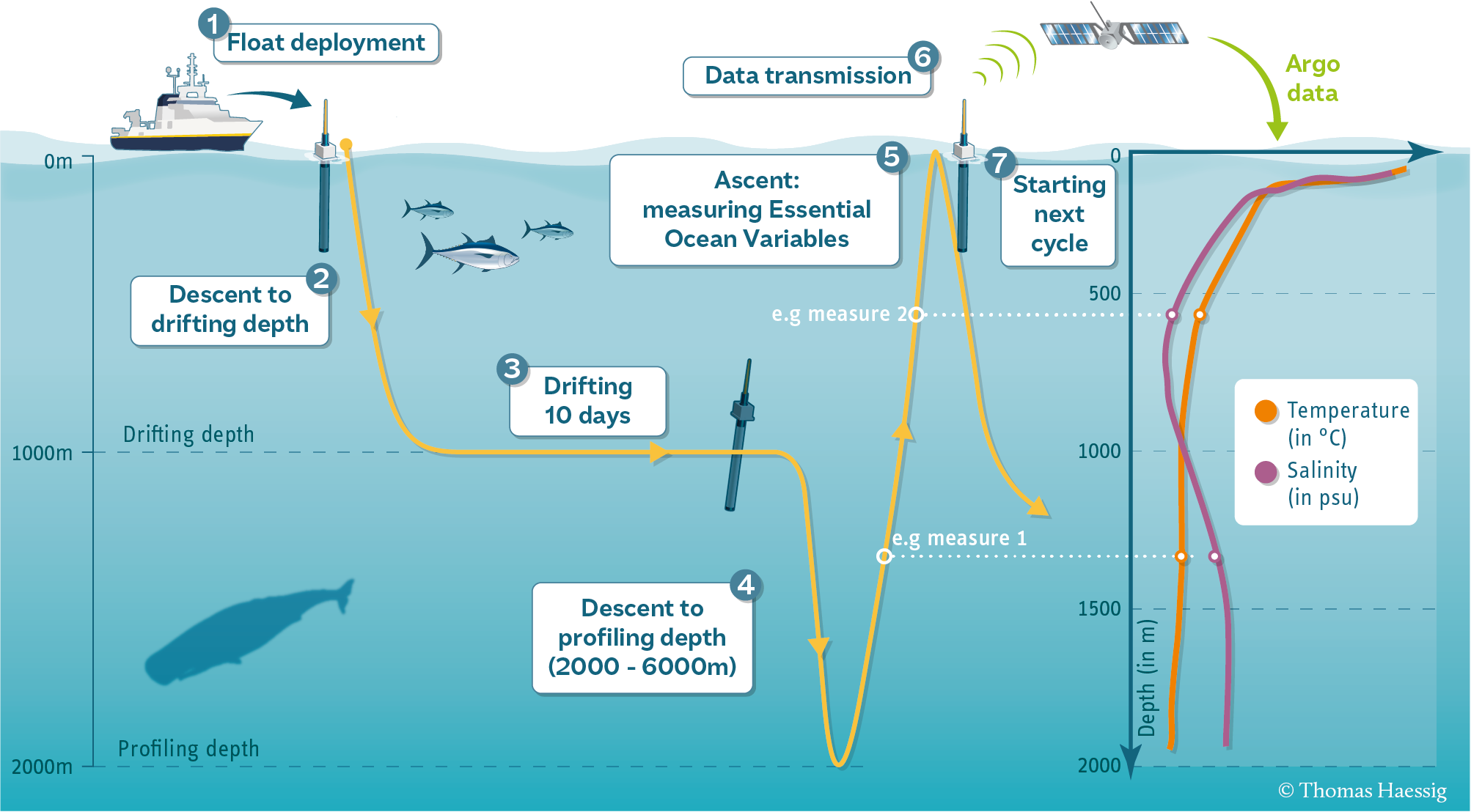
Each profile has a single latitude, longitude, and date associated with it, in addition to measurements at different levels.
Let’s start by using pooch to download the data files we need for this exercise. The following code will give you a list of .npy
files that you can open in the next step.
Note
Here we are passing a hash to the retrieve method from pooch
. Hash-based verification ensures that a file has not been corrupted by comparing the file’s hash value to a previously calculated value. If these values match, the file is presumed to be unmodified.
import pooch
url = "https://www.ldeo.columbia.edu/~rpa/float_data_4901412.zip"
files = pooch.retrieve(url, processor=pooch.Unzip(), known_hash="2a703c720302c682f1662181d329c9f22f9f10e1539dc2d6082160a469165009")
files.sort()
files
Downloading data from 'https://www.ldeo.columbia.edu/~rpa/float_data_4901412.zip' to file '/home/gisuser/.cache/pooch/7e6685dbe2a3c0b0870f770f3ef413d9-float_data_4901412.zip'.
Unzipping contents of '/home/gisuser/.cache/pooch/7e6685dbe2a3c0b0870f770f3ef413d9-float_data_4901412.zip' to '/home/gisuser/.cache/pooch/7e6685dbe2a3c0b0870f770f3ef413d9-float_data_4901412.zip.unzip'
['/home/gisuser/.cache/pooch/7e6685dbe2a3c0b0870f770f3ef413d9-float_data_4901412.zip.unzip/float_data/P.npy',
'/home/gisuser/.cache/pooch/7e6685dbe2a3c0b0870f770f3ef413d9-float_data_4901412.zip.unzip/float_data/S.npy',
'/home/gisuser/.cache/pooch/7e6685dbe2a3c0b0870f770f3ef413d9-float_data_4901412.zip.unzip/float_data/T.npy',
'/home/gisuser/.cache/pooch/7e6685dbe2a3c0b0870f770f3ef413d9-float_data_4901412.zip.unzip/float_data/date.npy',
'/home/gisuser/.cache/pooch/7e6685dbe2a3c0b0870f770f3ef413d9-float_data_4901412.zip.unzip/float_data/lat.npy',
'/home/gisuser/.cache/pooch/7e6685dbe2a3c0b0870f770f3ef413d9-float_data_4901412.zip.unzip/float_data/levels.npy',
'/home/gisuser/.cache/pooch/7e6685dbe2a3c0b0870f770f3ef413d9-float_data_4901412.zip.unzip/float_data/lon.npy']
We will manually load each of these variables into a numpy
array.
If this seems repetitive and inefficient, that’s the point!
numpy
itself is not meant for managing groups of inter-related arrays. That’s what xarray
is for!
P = np.load(files[0])
S = np.load(files[1])
T = np.load(files[2])
date = np.load(files[3])
lat = np.load(files[4])
levels = np.load(files[5])
lon = np.load(files[6])
Let’s look at the shape of some of the variables:
print("Salinity shape is:", S.shape)
print("Levels shape is:", levels.shape)
print("Date shape is:", date.shape)
Salinity shape is: (78, 75)
Levels shape is: (78,)
Date shape is: (75,)
As expected, Salinity has dimensions of levels x date
Let’s organize the data and coordinates of the salinity variable into a DataArray.
da_salinity = xr.DataArray(S, dims=['level', 'date'],
coords={'level': levels,
'date': date},)
da_salinity
<xarray.DataArray (level: 78, date: 75)> Size: 47kB array([[35.6389389 , 35.51495743, 35.57297134, ..., 35.82093811, 35.77793884, 35.66891098], [35.63393784, 35.5219574 , 35.57397079, ..., 35.81093216, 35.58389664, 35.66791153], [35.6819458 , 35.52595901, 35.57297134, ..., 35.79592896, 35.66290665, 35.66591263], ..., [34.91585922, 34.92390442, 34.92390442, ..., 34.93481064, 34.94081116, 34.94680786], [34.91585922, 34.92390442, 34.92190552, ..., 34.93280792, 34.93680954, 34.94380951], [34.91785812, 34.92390442, 34.92390442, ..., nan, 34.93680954, nan]]) Coordinates: * level (level) int64 624B 0 1 2 3 4 5 6 7 8 ... 69 70 71 72 73 74 75 76 77 * date (date) datetime64[ns] 600B 2012-07-13T22:33:06.019200 ... 2014-0...
da_salinity.plot()
<matplotlib.collections.QuadMesh at 0xffff58ab5d30>
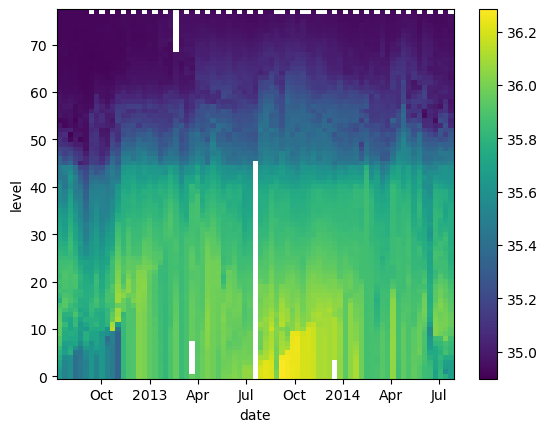
Since we are working with ocean data, it’s helpful to plot the level
in an increasing order from top to bottom:
da_salinity.plot(yincrease=False)
<matplotlib.collections.QuadMesh at 0xffff58874190>
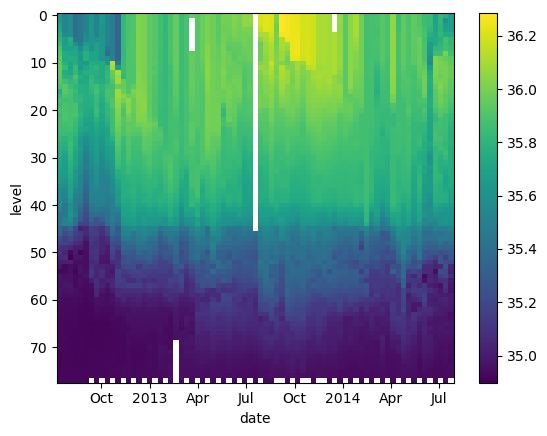
Attributes can be used to store metadata. What metadata should you store? The CF Conventions are a great resource for thinking about climate metadata. Below we define two of the required CF-conventions attributes.
da_salinity.attrs['units'] = 'PSU'
da_salinity.attrs['standard_name'] = 'sea_water_salinity'
da_salinity
<xarray.DataArray (level: 78, date: 75)> Size: 47kB array([[35.6389389 , 35.51495743, 35.57297134, ..., 35.82093811, 35.77793884, 35.66891098], [35.63393784, 35.5219574 , 35.57397079, ..., 35.81093216, 35.58389664, 35.66791153], [35.6819458 , 35.52595901, 35.57297134, ..., 35.79592896, 35.66290665, 35.66591263], ..., [34.91585922, 34.92390442, 34.92390442, ..., 34.93481064, 34.94081116, 34.94680786], [34.91585922, 34.92390442, 34.92190552, ..., 34.93280792, 34.93680954, 34.94380951], [34.91785812, 34.92390442, 34.92390442, ..., nan, 34.93680954, nan]]) Coordinates: * level (level) int64 624B 0 1 2 3 4 5 6 7 8 ... 69 70 71 72 73 74 75 76 77 * date (date) datetime64[ns] 600B 2012-07-13T22:33:06.019200 ... 2014-0... Attributes: units: PSU standard_name: sea_water_salinity
Now if we plot the data again, the name and units are automatically attached to the figure.
da_salinity.plot()
<matplotlib.collections.QuadMesh at 0xffff58732ad0>
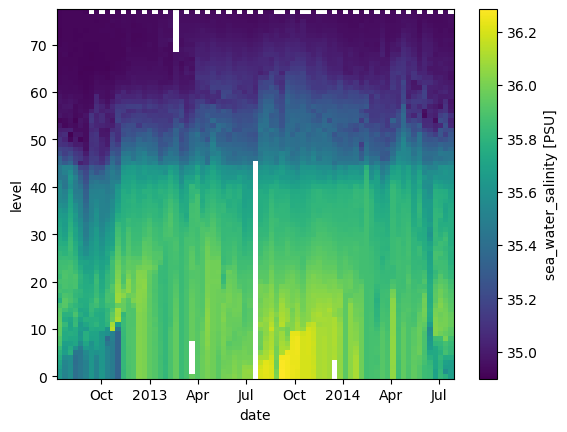
20.1.3. Datasets#
A Dataset
holds many DataArray
s which potentially can share coordinates. In analogy to pandas:
pandas.Series : pandas.Dataframe :: xarray.DataArray : xarray.Dataset
Constructing Dataset
s manually is a bit more involved in terms of syntax. The Dataset
constructor takes three arguments:
data_vars
should be a dictionary with each key as the name of the variable and each value as one of:A
DataArray
or VariableA tuple of the form
(dims, data[, attrs])
, which is converted into arguments for VariableA
pandas
object, which is converted into aDataArray
A 1D array or list, which is interpreted as values for a one dimensional coordinate variable along the same dimension as it’s name
coords
should be a dictionary of the same form asdata_vars
.attrs
should be a dictionary.
Let’s put together a Dataset with temperature, salinity and pressure all together
argo = xr.Dataset(
data_vars={
'salinity': (('level', 'date'), S),
'temperature': (('level', 'date'), T),
'pressure': (('level', 'date'), P)
},
coords={
'level': levels,
'date': date
}
)
argo
<xarray.Dataset> Size: 142kB Dimensions: (level: 78, date: 75) Coordinates: * level (level) int64 624B 0 1 2 3 4 5 6 7 ... 70 71 72 73 74 75 76 77 * date (date) datetime64[ns] 600B 2012-07-13T22:33:06.019200 ... 20... Data variables: salinity (level, date) float64 47kB 35.64 35.51 35.57 ... nan 34.94 nan temperature (level, date) float64 47kB 18.97 18.44 19.1 ... nan 3.714 nan pressure (level, date) float64 47kB 6.8 6.1 6.5 5.0 ... nan 2e+03 nan
What about lon and lat? We forgot them in the creation process, but we can add them after the fact.
argo.coords['lon'] = lon
argo
<xarray.Dataset> Size: 142kB Dimensions: (level: 78, date: 75, lon: 75) Coordinates: * level (level) int64 624B 0 1 2 3 4 5 6 7 ... 70 71 72 73 74 75 76 77 * date (date) datetime64[ns] 600B 2012-07-13T22:33:06.019200 ... 20... * lon (lon) float64 600B -39.13 -37.28 -36.9 ... -33.83 -34.11 -34.38 Data variables: salinity (level, date) float64 47kB 35.64 35.51 35.57 ... nan 34.94 nan temperature (level, date) float64 47kB 18.97 18.44 19.1 ... nan 3.714 nan pressure (level, date) float64 47kB 6.8 6.1 6.5 5.0 ... nan 2e+03 nan
That was not quite right…we want lon to have dimension date
:
del argo['lon']
argo.coords['lon'] = ('date', lon)
argo.coords['lat'] = ('date', lat)
argo
<xarray.Dataset> Size: 143kB Dimensions: (level: 78, date: 75) Coordinates: * level (level) int64 624B 0 1 2 3 4 5 6 7 ... 70 71 72 73 74 75 76 77 * date (date) datetime64[ns] 600B 2012-07-13T22:33:06.019200 ... 20... lon (date) float64 600B -39.13 -37.28 -36.9 ... -34.11 -34.38 lat (date) float64 600B 47.19 46.72 46.45 ... 42.6 42.46 42.38 Data variables: salinity (level, date) float64 47kB 35.64 35.51 35.57 ... nan 34.94 nan temperature (level, date) float64 47kB 18.97 18.44 19.1 ... nan 3.714 nan pressure (level, date) float64 47kB 6.8 6.1 6.5 5.0 ... nan 2e+03 nan
20.1.4. Coordinates vs. Data Variables#
Data variables can be modified through arithmetic operations or other functions. Coordinates are always kept the same.
argo * 10000
<xarray.Dataset> Size: 143kB Dimensions: (level: 78, date: 75) Coordinates: * level (level) int64 624B 0 1 2 3 4 5 6 7 ... 70 71 72 73 74 75 76 77 * date (date) datetime64[ns] 600B 2012-07-13T22:33:06.019200 ... 20... lon (date) float64 600B -39.13 -37.28 -36.9 ... -34.11 -34.38 lat (date) float64 600B 47.19 46.72 46.45 ... 42.6 42.46 42.38 Data variables: salinity (level, date) float64 47kB 3.564e+05 3.551e+05 ... nan temperature (level, date) float64 47kB 1.897e+05 1.844e+05 ... nan pressure (level, date) float64 47kB 6.8e+04 6.1e+04 ... 2e+07 nan
Clearly lon and lat are coordinates and not data variables.
The bold font in the representation above indicates that level
and date
are “dimension coordinates” (they describe the coordinates associated with data variable axes) while lon
and lat
are “non-dimension coordinates”. We can make any variable a non-dimension coordinate (using set_coords
).
20.2. Selecting Data (Indexing)#
We can always use regular numpy
indexing and slicing on DataArray
s.
argo.salinity.shape
(78, 75)
argo.salinity[2]
<xarray.DataArray 'salinity' (date: 75)> Size: 600B array([35.6819458 , 35.52595901, 35.57297134, 35.40494537, 35.45091629, 35.50192261, 35.62397766, 35.51696014, 35.62797546, 35.52292252, 35.47383118, 35.33785629, 35.81896591, 35.88694 , 35.90187836, 36.02391815, 36.00475693, 35.94187927, 35.91583252, 35.86392212, 35.81995392, 35.88601303, 35.95079422, 35.84091568, 35.87992477, nan, 35.92179108, 35.96979141, 36.0008316 , 35.98083115, 35.92887878, 35.98091888, 35.9838829 , 36.01884842, 35.99092484, 36.04689026, 36.04185867, nan, 36.19193268, 36.22789764, 36.20986557, 35.97589874, 36.2779007 , 36.25889969, 36.2418251 , 36.23685837, 36.19781876, 36.19785309, 36.17692184, 36.1048851 , 36.11392212, 36.09080505, nan, 36.05675888, 35.93374634, 36.04291534, 36.10183716, 35.97779083, 35.86592102, 35.87791824, 35.88392258, 35.92078781, 35.88601303, 36.05178833, 35.85883713, 35.94878769, 35.8938446 , 35.94379425, 35.90884018, 35.84893036, 35.83496857, 35.71691132, 35.79592896, 35.66290665, 35.66591263]) Coordinates: level int64 8B 2 * date (date) datetime64[ns] 600B 2012-07-13T22:33:06.019200 ... 2014-0... lon (date) float64 600B -39.13 -37.28 -36.9 ... -33.83 -34.11 -34.38 lat (date) float64 600B 47.19 46.72 46.45 46.23 ... 42.6 42.46 42.38
argo.salinity[2].plot()
[<matplotlib.lines.Line2D at 0xffff58639950>]
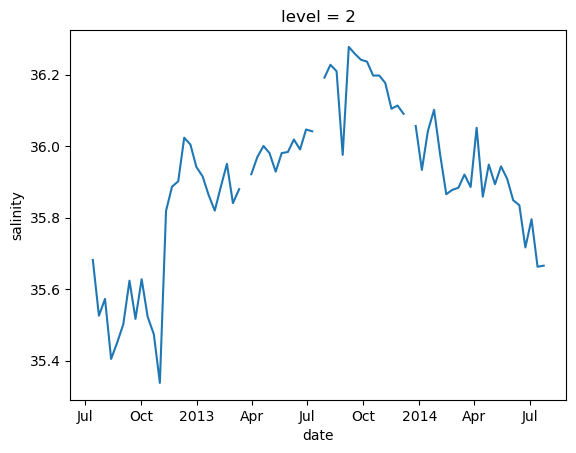
argo.salinity[:, 10]
<xarray.DataArray 'salinity' (level: 78)> Size: 624B array([35.47483063, 35.47483063, 35.47383118, 35.47383118, 35.47383118, 35.47483063, 35.48183441, 35.47983551, 35.4948349 , 35.51083755, 36.13380051, 36.09579849, 35.95479965, 35.93979645, 35.8958931 , 35.86388397, 35.87788773, 35.88188934, 35.90379333, 35.9067955 , 35.86588669, 35.8588829 , 35.86088181, 35.85188293, 35.85788345, 35.82787323, 35.78786469, 35.73185349, 35.69784927, 35.67684174, 35.677845 , 35.65784073, 35.64083481, 35.6238327 , 35.59682846, 35.57782364, 35.56182098, 35.55781937, 35.52181625, 35.49881363, 35.51381302, 35.49981308, 35.47280884, 35.47880936, 35.44780731, 35.39379501, 35.35879135, 35.28778076, 35.21878052, 35.20677567, 35.17777252, 35.15076828, 35.07276535, 35.01475525, 34.9797554 , 35.0117569 , 35.03975677, 35.05575562, 35.00975037, 34.96175385, 34.96775055, 34.95075226, 34.93775177, 34.93375015, 34.93775558, 34.9247551 , 34.92175674, 34.91975403, 34.91975403, 34.91975403, 34.92176056, 34.92375946, 34.92575836, 34.92575836, 34.92475891, 34.93076324, 34.92176437, nan]) Coordinates: * level (level) int64 624B 0 1 2 3 4 5 6 7 8 ... 69 70 71 72 73 74 75 76 77 date datetime64[ns] 8B 2012-10-22T02:50:32.006400 lon float64 8B -32.97 lat float64 8B 44.13
argo.salinity[:, 10:11].plot()
[<matplotlib.lines.Line2D at 0xffff586ab890>]
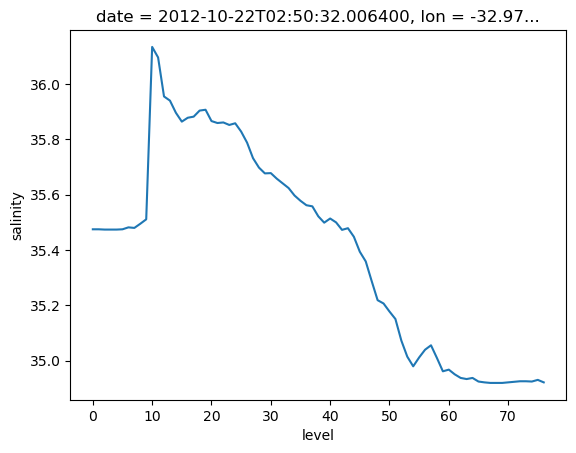
However, it is often more powerful to use xarray
’s .sel()
method to use label-based indexing.
argo.salinity.sel(level=[2, 5])
<xarray.DataArray 'salinity' (level: 2, date: 75)> Size: 1kB array([[35.6819458 , 35.52595901, 35.57297134, 35.40494537, 35.45091629, 35.50192261, 35.62397766, 35.51696014, 35.62797546, 35.52292252, 35.47383118, 35.33785629, 35.81896591, 35.88694 , 35.90187836, 36.02391815, 36.00475693, 35.94187927, 35.91583252, 35.86392212, 35.81995392, 35.88601303, 35.95079422, 35.84091568, 35.87992477, nan, 35.92179108, 35.96979141, 36.0008316 , 35.98083115, 35.92887878, 35.98091888, 35.9838829 , 36.01884842, 35.99092484, 36.04689026, 36.04185867, nan, 36.19193268, 36.22789764, 36.20986557, 35.97589874, 36.2779007 , 36.25889969, 36.2418251 , 36.23685837, 36.19781876, 36.19785309, 36.17692184, 36.1048851 , 36.11392212, 36.09080505, nan, 36.05675888, 35.93374634, 36.04291534, 36.10183716, 35.97779083, 35.86592102, 35.87791824, 35.88392258, 35.92078781, 35.88601303, 36.05178833, 35.85883713, 35.94878769, 35.8938446 , 35.94379425, 35.90884018, 35.84893036, 35.83496857, 35.71691132, 35.79592896, 35.66290665, 35.66591263], [35.7889595 , 35.67097855, 35.77500153, 35.4429512 , 35.44491196, 35.55392838, 35.62497711, 35.59697342, 35.62897873, 35.52292252, 35.47483063, 35.33785629, 35.81996536, 35.88993835, 35.90187836, 36.02191925, 36.00475693, 35.93987656, 35.91583633, 35.86492538, 35.81995773, 35.8870163 , 35.95079422, 35.84191513, 35.87992477, nan, 35.92179108, 35.96479034, 36.00683594, 35.98083115, 35.9368782 , 35.99091721, 35.962883 , 35.98684311, 35.97792435, 35.98988724, 35.97084808, nan, 36.0339241 , 36.09988785, 36.15286255, 35.98789215, 36.11489487, 36.24489975, 36.24282455, 36.24985886, 36.19781876, 36.19885254, 36.17592239, 36.10388565, 36.11192322, 36.09080505, 36.11088181, 36.05976105, 35.93475342, 36.04491425, 36.08283615, 35.97679138, 35.86592102, 35.87791824, 35.88392258, 35.92078781, 35.88500977, 36.0527916 , 35.87383652, 35.9487915 , 35.8938446 , 35.9407959 , 35.97483444, 35.87393188, 35.73094177, 35.73391342, 35.77991486, 35.87294388, 35.8139267 ]]) Coordinates: * level (level) int64 16B 2 5 * date (date) datetime64[ns] 600B 2012-07-13T22:33:06.019200 ... 2014-0... lon (date) float64 600B -39.13 -37.28 -36.9 ... -33.83 -34.11 -34.38 lat (date) float64 600B 47.19 46.72 46.45 46.23 ... 42.6 42.46 42.38
argo.salinity.sel(level=2)
<xarray.DataArray 'salinity' (date: 75)> Size: 600B array([35.6819458 , 35.52595901, 35.57297134, 35.40494537, 35.45091629, 35.50192261, 35.62397766, 35.51696014, 35.62797546, 35.52292252, 35.47383118, 35.33785629, 35.81896591, 35.88694 , 35.90187836, 36.02391815, 36.00475693, 35.94187927, 35.91583252, 35.86392212, 35.81995392, 35.88601303, 35.95079422, 35.84091568, 35.87992477, nan, 35.92179108, 35.96979141, 36.0008316 , 35.98083115, 35.92887878, 35.98091888, 35.9838829 , 36.01884842, 35.99092484, 36.04689026, 36.04185867, nan, 36.19193268, 36.22789764, 36.20986557, 35.97589874, 36.2779007 , 36.25889969, 36.2418251 , 36.23685837, 36.19781876, 36.19785309, 36.17692184, 36.1048851 , 36.11392212, 36.09080505, nan, 36.05675888, 35.93374634, 36.04291534, 36.10183716, 35.97779083, 35.86592102, 35.87791824, 35.88392258, 35.92078781, 35.88601303, 36.05178833, 35.85883713, 35.94878769, 35.8938446 , 35.94379425, 35.90884018, 35.84893036, 35.83496857, 35.71691132, 35.79592896, 35.66290665, 35.66591263]) Coordinates: level int64 8B 2 * date (date) datetime64[ns] 600B 2012-07-13T22:33:06.019200 ... 2014-0... lon (date) float64 600B -39.13 -37.28 -36.9 ... -33.83 -34.11 -34.38 lat (date) float64 600B 47.19 46.72 46.45 46.23 ... 42.6 42.46 42.38
Under the hood, this method is powered by using pandas
’s powerful Index objects which means this method uses pandas
’s (well documented) logic for indexing. For example, you can use string shortcuts for datetime indexes (e.g., ‘2000-01’ to select all values in January 2000). It also means that slices are treated as inclusive of both the start and stop values, unlike normal Python indexing.
argo.salinity.sel(level=2).plot()
[<matplotlib.lines.Line2D at 0xffff5852a490>]
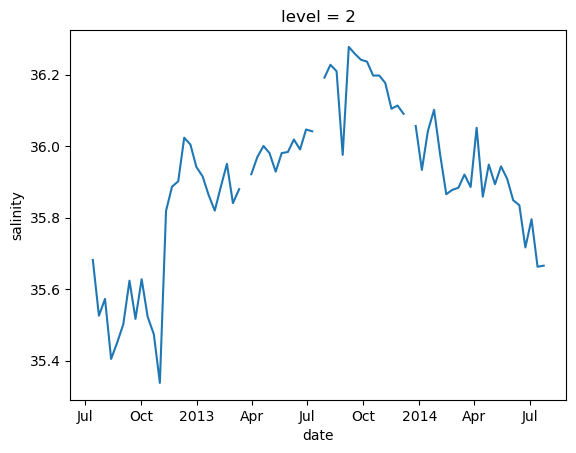
argo.salinity.sel(date='2012-10-22')
<xarray.DataArray 'salinity' (level: 78, date: 1)> Size: 624B array([[35.47483063], [35.47483063], [35.47383118], [35.47383118], [35.47383118], [35.47483063], [35.48183441], [35.47983551], [35.4948349 ], [35.51083755], [36.13380051], [36.09579849], [35.95479965], [35.93979645], [35.8958931 ], [35.86388397], [35.87788773], [35.88188934], [35.90379333], [35.9067955 ], ... [35.00975037], [34.96175385], [34.96775055], [34.95075226], [34.93775177], [34.93375015], [34.93775558], [34.9247551 ], [34.92175674], [34.91975403], [34.91975403], [34.91975403], [34.92176056], [34.92375946], [34.92575836], [34.92575836], [34.92475891], [34.93076324], [34.92176437], [ nan]]) Coordinates: * level (level) int64 624B 0 1 2 3 4 5 6 7 8 ... 69 70 71 72 73 74 75 76 77 * date (date) datetime64[ns] 8B 2012-10-22T02:50:32.006400 lon (date) float64 8B -32.97 lat (date) float64 8B 44.13
We can improve the plot by using the yincrease
argument, and setting the variable that will be plotted on y
axis:
argo.salinity.sel(date='2012-10-22').plot(y='level', yincrease=False)
[<matplotlib.lines.Line2D at 0xffff585cc190>]
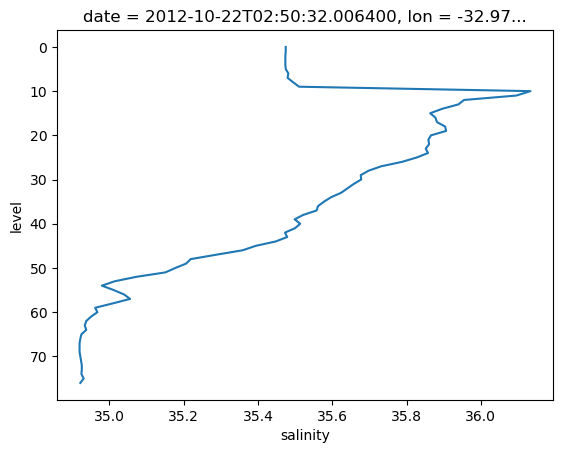
.sel()
also supports slicing.
argo.salinity.sel(date=slice('2012-10-01', '2012-12-01'))
<xarray.DataArray 'salinity' (level: 78, date: 7)> Size: 4kB array([[35.63097763, 35.52592468, 35.47483063, 35.33785629, 35.81896591, 35.8889389 , 35.90187836], [35.63097763, 35.52292252, 35.47483063, 35.33685684, 35.81796646, 35.88793945, 35.90187836], [35.62797546, 35.52292252, 35.47383118, 35.33785629, 35.81896591, 35.88694 , 35.90187836], [35.62697601, 35.52192307, 35.47383118, 35.33785629, 35.81896591, 35.89193726, 35.90187836], [35.62797546, 35.52192307, 35.47383118, 35.33785629, 35.81996536, 35.88993835, 35.90187836], [35.62897873, 35.52292252, 35.47483063, 35.33785629, 35.81996536, 35.88993835, 35.90187836], [35.62997818, 35.51892471, 35.48183441, 35.33785629, 35.81996536, 35.88993835, 35.90187836], [35.63197708, 35.44991302, 35.47983551, 35.33785629, 35.81996536, 35.89683914, 35.90187836], [35.63097763, 35.38090134, 35.4948349 , 35.33785629, 35.81896591, 35.89583969, 35.90187836], [35.62697601, 35.58792114, 35.51083755, 35.33985519, 35.82497025, 35.89683914, 35.90187836], ... [34.91690445, 34.92385483, 34.91975403, 34.91980362, 34.92385483, 34.93680573, 34.93885422], [34.92190552, 34.92485428, 34.91975403, 34.92080688, 34.92485428, 34.94480515, 34.9328537 ], [34.92390442, 34.92285538, 34.92176056, 34.92280579, 34.92985535, 34.93280411, 34.92785645], [34.92390442, 34.92385483, 34.92375946, 34.92480469, 34.92685318, 34.93780899, 34.92485428], [34.92390442, 34.92285538, 34.92575836, 34.92181015, 34.92085648, 34.93680954, 34.92385483], [34.92590332, 34.9288559 , 34.92575836, 34.92181015, 34.92685318, 34.93481064, 34.92585373], [34.92490387, 34.92785645, 34.92475891, 34.92781067, 34.93385696, 34.93380737, 34.92385864], [34.92190552, 34.92385864, 34.93076324, 34.9268074 , 34.93585968, 34.93481064, 34.92985916], [34.92090607, 34.92185974, 34.92176437, 34.9228096 , 34.93285751, 34.93180847, 34.92786026], [ nan, 34.91985703, nan, 34.92181015, nan, 34.92181015, nan]]) Coordinates: * level (level) int64 624B 0 1 2 3 4 5 6 7 8 ... 69 70 71 72 73 74 75 76 77 * date (date) datetime64[ns] 56B 2012-10-02T03:00:17.971200 ... 2012-12... lon (date) float64 56B -34.46 -33.78 -32.97 -32.55 -32.43 -32.29 -32.17 lat (date) float64 56B 44.96 44.68 44.13 43.64 43.07 42.66 42.51
argo.salinity.sel(date=slice('2012-10-01', '2012-12-01')).plot()
<matplotlib.collections.QuadMesh at 0xffff58422d50>
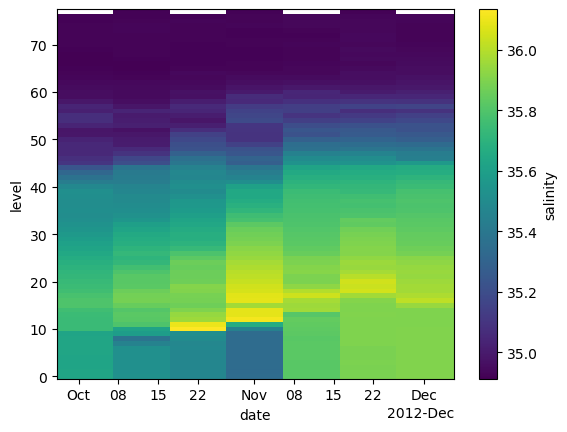
.sel()
also works on the whole Dataset
argo.sel(date='2012-10-22')
<xarray.Dataset> Size: 3kB Dimensions: (level: 78, date: 1) Coordinates: * level (level) int64 624B 0 1 2 3 4 5 6 7 ... 70 71 72 73 74 75 76 77 * date (date) datetime64[ns] 8B 2012-10-22T02:50:32.006400 lon (date) float64 8B -32.97 lat (date) float64 8B 44.13 Data variables: salinity (level, date) float64 624B 35.47 35.47 35.47 ... 34.92 nan temperature (level, date) float64 624B 17.13 17.13 17.13 ... 3.639 nan pressure (level, date) float64 624B 6.4 10.3 15.4 ... 1.951e+03 nan
argo.sel(date = '2012-12-22')
<xarray.Dataset> Size: 624B Dimensions: (level: 78, date: 0) Coordinates: * level (level) int64 624B 0 1 2 3 4 5 6 7 ... 70 71 72 73 74 75 76 77 * date (date) datetime64[ns] 0B lon (date) float64 0B lat (date) float64 0B Data variables: salinity (level, date) float64 0B temperature (level, date) float64 0B pressure (level, date) float64 0B
20.3. Computation#
xarray
DataArrays
and Datasets
work seamlessly with arithmetic operators and numpy
array functions.
temp_kelvin = argo.temperature + 273.15
temp_kelvin.plot(yincrease=False)
<matplotlib.collections.QuadMesh at 0xffff58319810>
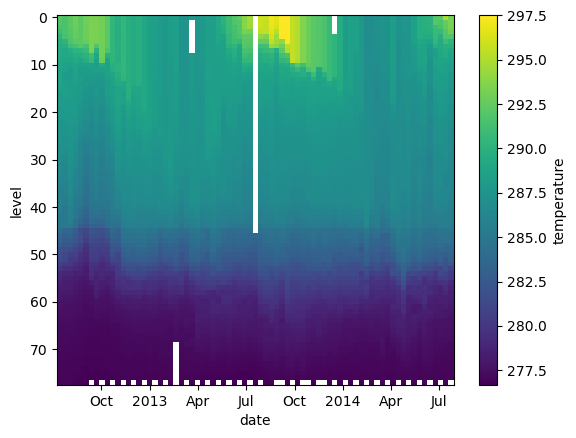
We can also combine multiple xarray
Dataset
s in arithmetic operations
g = 9.8
buoyancy = g * (2e-4 * argo.temperature - 7e-4 * argo.salinity)
buoyancy.plot(yincrease=False)
<matplotlib.collections.QuadMesh at 0xffff58200e10>
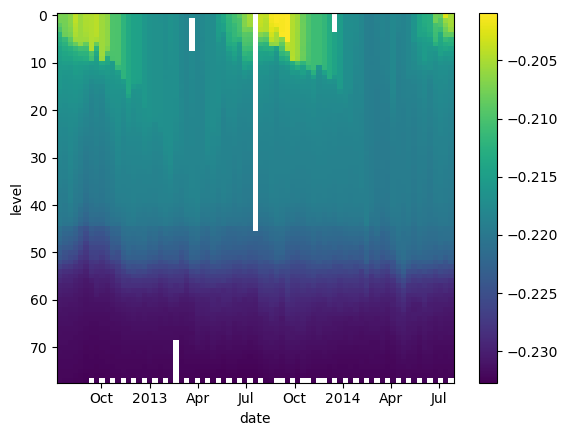
20.4. Broadcasting, Aligment, and Combining Data#
20.4.1. Broadcasting#
Broadcasting arrays in numpy
is a nightmare. It is much easier when the data axes are labeled!
This is a useless calculation, but it illustrates how performing an operation on arrays with different coordinates will result in automatic broadcasting.
argo.level
<xarray.DataArray 'level' (level: 78)> Size: 624B array([ 0, 1, 2, 3, 4, 5, 6, 7, 8, 9, 10, 11, 12, 13, 14, 15, 16, 17, 18, 19, 20, 21, 22, 23, 24, 25, 26, 27, 28, 29, 30, 31, 32, 33, 34, 35, 36, 37, 38, 39, 40, 41, 42, 43, 44, 45, 46, 47, 48, 49, 50, 51, 52, 53, 54, 55, 56, 57, 58, 59, 60, 61, 62, 63, 64, 65, 66, 67, 68, 69, 70, 71, 72, 73, 74, 75, 76, 77]) Coordinates: * level (level) int64 624B 0 1 2 3 4 5 6 7 8 ... 69 70 71 72 73 74 75 76 77
argo.lat
<xarray.DataArray 'lat' (date: 75)> Size: 600B array([47.187, 46.716, 46.45 , 46.23 , 45.459, 44.833, 44.452, 44.839, 44.956, 44.676, 44.13 , 43.644, 43.067, 42.662, 42.513, 42.454, 42.396, 42.256, 42.089, 41.944, 41.712, 41.571, 41.596, 41.581, 41.351, 41.032, 40.912, 40.792, 40.495, 40.383, 40.478, 40.672, 41.032, 40.864, 40.651, 40.425, 40.228, 40.197, 40.483, 40.311, 40.457, 40.463, 40.164, 40.047, 39.963, 40.122, 40.57 , 40.476, 40.527, 40.589, 40.749, 40.993, 41.162, 41.237, 41.448, 41.65 , 42.053, 42.311, 42.096, 41.683, 41.661, 41.676, 42.018, 42.395, 42.532, 42.558, 42.504, 42.63 , 42.934, 42.952, 42.777, 42.722, 42.601, 42.457, 42.379]) Coordinates: * date (date) datetime64[ns] 600B 2012-07-13T22:33:06.019200 ... 2014-0... lon (date) float64 600B -39.13 -37.28 -36.9 ... -33.83 -34.11 -34.38 lat (date) float64 600B 47.19 46.72 46.45 46.23 ... 42.6 42.46 42.38
level_times_lat = argo.level * argo.lat
level_times_lat
<xarray.DataArray (level: 78, date: 75)> Size: 47kB array([[ 0. , 0. , 0. , ..., 0. , 0. , 0. ], [ 47.187, 46.716, 46.45 , ..., 42.601, 42.457, 42.379], [ 94.374, 93.432, 92.9 , ..., 85.202, 84.914, 84.758], ..., [3539.025, 3503.7 , 3483.75 , ..., 3195.075, 3184.275, 3178.425], [3586.212, 3550.416, 3530.2 , ..., 3237.676, 3226.732, 3220.804], [3633.399, 3597.132, 3576.65 , ..., 3280.277, 3269.189, 3263.183]]) Coordinates: * level (level) int64 624B 0 1 2 3 4 5 6 7 8 ... 69 70 71 72 73 74 75 76 77 * date (date) datetime64[ns] 600B 2012-07-13T22:33:06.019200 ... 2014-0... lon (date) float64 600B -39.13 -37.28 -36.9 ... -33.83 -34.11 -34.38 lat (date) float64 600B 47.19 46.72 46.45 46.23 ... 42.6 42.46 42.38
level_times_lat.plot()
<matplotlib.collections.QuadMesh at 0xffff582cad50>
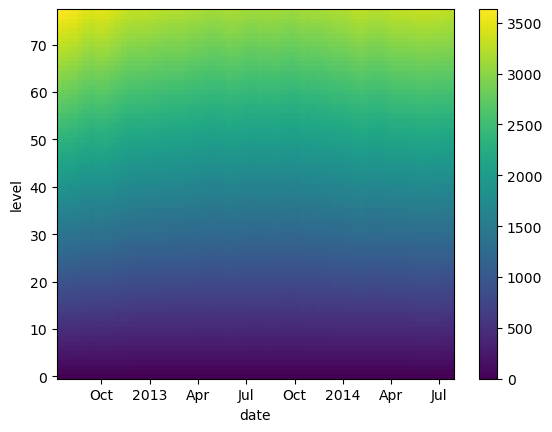
20.4.2. Alignment#
If you try to perform operations on DataArray
s that share a dimension name, xarray
will try to align them first.
This works nearly identically to pandas
, except that there can be multiple dimensions to align over.
To see how alignment works, we will create some subsets of our original data.
sa_surf = argo.salinity.isel(level=slice(0, 20))
sa_mid = argo.salinity.isel(level=slice(10, 30))
By default, when we combine multiple arrays in mathematical operations, xarray
performs an “inner join”.
(sa_surf * sa_mid).level
<xarray.DataArray 'level' (level: 10)> Size: 80B array([10, 11, 12, 13, 14, 15, 16, 17, 18, 19]) Coordinates: * level (level) int64 80B 10 11 12 13 14 15 16 17 18 19
We can override this behavior by manually aligning the data:
sa_surf_outer, sa_mid_outer = xr.align(sa_surf, sa_mid, join='outer')
sa_surf_outer
<xarray.DataArray 'salinity' (level: 30, date: 75)> Size: 18kB array([[35.6389389 , 35.51495743, 35.57297134, ..., 35.82093811, 35.77793884, 35.66891098], [35.63393784, 35.5219574 , 35.57397079, ..., 35.81093216, 35.58389664, 35.66791153], [35.6819458 , 35.52595901, 35.57297134, ..., 35.79592896, 35.66290665, 35.66591263], ..., [ nan, nan, nan, ..., nan, nan, nan], [ nan, nan, nan, ..., nan, nan, nan], [ nan, nan, nan, ..., nan, nan, nan]]) Coordinates: * level (level) int64 240B 0 1 2 3 4 5 6 7 8 ... 21 22 23 24 25 26 27 28 29 * date (date) datetime64[ns] 600B 2012-07-13T22:33:06.019200 ... 2014-0... lon (date) float64 600B -39.13 -37.28 -36.9 ... -33.83 -34.11 -34.38 lat (date) float64 600B 47.19 46.72 46.45 46.23 ... 42.6 42.46 42.38
As we can see, missing data (NaNs) have been filled in where the array was extended.
From the documentation: Missing values (if join != 'inner'
) are filled with fill_value
. The default fill value is NaN.
sa_surf_outer.plot(yincrease=False)
<matplotlib.collections.QuadMesh at 0xffff581b1090>
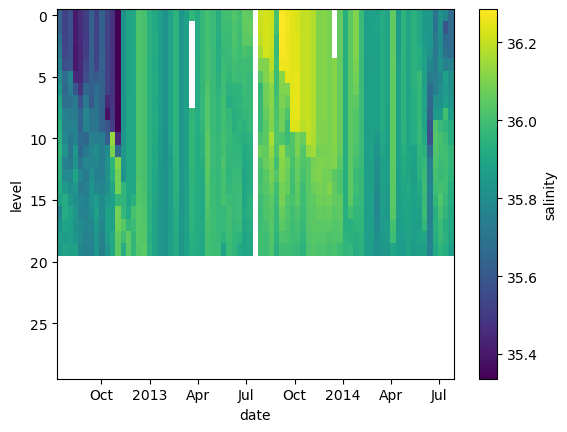
We can also use join='right'
and join='left'
.
If we run our multiplication on the aligned data:
(sa_surf_outer * sa_mid_outer).level
<xarray.DataArray 'level' (level: 30)> Size: 240B array([ 0, 1, 2, 3, 4, 5, 6, 7, 8, 9, 10, 11, 12, 13, 14, 15, 16, 17, 18, 19, 20, 21, 22, 23, 24, 25, 26, 27, 28, 29]) Coordinates: * level (level) int64 240B 0 1 2 3 4 5 6 7 8 ... 21 22 23 24 25 26 27 28 29
20.4.3. Combing Data: Concat and Merge#
The ability to combine many smaller arrays into a single big Dataset
is one of the main advantages of xarray
.
To take advantage of this, we need to learn two operations that help us combine data:
xr.contact
: to concatenate multiple arrays into one bigger array along their dimensions.xr.merge
: to combine multiple different arrays into aDataset
.
First let’s look at concat
. Let’s re-combine the subset data from the previous step.
sa_surf_mid = xr.concat([sa_surf, sa_mid], dim='level')
sa_surf_mid
<xarray.DataArray 'salinity' (level: 40, date: 75)> Size: 24kB array([[35.6389389 , 35.51495743, 35.57297134, ..., 35.82093811, 35.77793884, 35.66891098], [35.63393784, 35.5219574 , 35.57397079, ..., 35.81093216, 35.58389664, 35.66791153], [35.6819458 , 35.52595901, 35.57297134, ..., 35.79592896, 35.66290665, 35.66591263], ..., [35.78895187, 35.7829895 , 35.85100555, ..., 35.84291458, 35.81891251, 35.7779007 ], [35.76794815, 35.75598526, 35.84500504, ..., 35.84891891, 35.83391571, 35.76390076], [35.75194168, 35.71097565, 35.83100128, ..., 35.80690765, 35.85292053, 35.75489807]]) Coordinates: * level (level) int64 320B 0 1 2 3 4 5 6 7 8 ... 21 22 23 24 25 26 27 28 29 * date (date) datetime64[ns] 600B 2012-07-13T22:33:06.019200 ... 2014-0... lon (date) float64 600B -39.13 -37.28 -36.9 ... -33.83 -34.11 -34.38 lat (date) float64 600B 47.19 46.72 46.45 46.23 ... 42.6 42.46 42.38
Warning
xarray
will not check the values of the coordinates before concat
. It will just stick everything together into a new array.
In this case, we had overlapping data. We can see this by looking at the level
coordinate.
sa_surf_mid.level
<xarray.DataArray 'level' (level: 40)> Size: 320B array([ 0, 1, 2, 3, 4, 5, 6, 7, 8, 9, 10, 11, 12, 13, 14, 15, 16, 17, 18, 19, 10, 11, 12, 13, 14, 15, 16, 17, 18, 19, 20, 21, 22, 23, 24, 25, 26, 27, 28, 29]) Coordinates: * level (level) int64 320B 0 1 2 3 4 5 6 7 8 ... 21 22 23 24 25 26 27 28 29
sa_surf_mid.sel(level=11)
<xarray.DataArray 'salinity' (level: 2, date: 75)> Size: 1kB array([[35.95887756, 35.82500458, 35.70597839, 35.8350029 , 35.64592743, 35.71894073, 35.76799011, 35.80199432, 35.7379837 , 35.79395294, 36.09579849, 35.6628952 , 35.83797073, 35.89783859, 35.89887619, 36.02391815, 36.00475693, 35.94387817, 35.90093613, 35.85692215, 35.81895828, 35.86300659, 35.94979477, 35.84291458, 35.87992477, 35.95978928, 35.92179108, 35.94578934, 36.04083633, 35.98183441, 35.96687317, 35.99791718, 35.96387863, 35.9588356 , 35.95691681, 35.91187668, 35.93383408, nan, 36.06692123, 36.05187607, 36.12983704, 36.01087952, 36.13287735, 36.02987671, 36.01980209, 36.09484482, 36.02880096, 36.13484573, 36.15492249, 36.08387756, 36.09292221, 36.09080505, 36.10988235, 36.05976105, 35.9327507 , 36.04291534, 36.01783371, 35.97879028, 35.86592102, 35.87792206, 35.82791138, 35.89588547, 35.86800766, 36.05178833, 35.87783432, 35.9337883 , 35.8938446 , 35.88488388, 35.95883179, 35.94283676, 35.67092896, 36.02183533, 35.99683762, 35.9948349 , 35.96183395], [35.95887756, 35.82500458, 35.70597839, 35.8350029 , 35.64592743, 35.71894073, 35.76799011, 35.80199432, 35.7379837 , 35.79395294, 36.09579849, 35.6628952 , 35.83797073, 35.89783859, 35.89887619, 36.02391815, 36.00475693, 35.94387817, 35.90093613, 35.85692215, 35.81895828, 35.86300659, 35.94979477, 35.84291458, 35.87992477, 35.95978928, 35.92179108, 35.94578934, 36.04083633, 35.98183441, 35.96687317, 35.99791718, 35.96387863, 35.9588356 , 35.95691681, 35.91187668, 35.93383408, nan, 36.06692123, 36.05187607, 36.12983704, 36.01087952, 36.13287735, 36.02987671, 36.01980209, 36.09484482, 36.02880096, 36.13484573, 36.15492249, 36.08387756, 36.09292221, 36.09080505, 36.10988235, 36.05976105, 35.9327507 , 36.04291534, 36.01783371, 35.97879028, 35.86592102, 35.87792206, 35.82791138, 35.89588547, 35.86800766, 36.05178833, 35.87783432, 35.9337883 , 35.8938446 , 35.88488388, 35.95883179, 35.94283676, 35.67092896, 36.02183533, 35.99683762, 35.9948349 , 35.96183395]]) Coordinates: * level (level) int64 16B 11 11 * date (date) datetime64[ns] 600B 2012-07-13T22:33:06.019200 ... 2014-0... lon (date) float64 600B -39.13 -37.28 -36.9 ... -33.83 -34.11 -34.38 lat (date) float64 600B 47.19 46.72 46.45 46.23 ... 42.6 42.46 42.38
plt.plot(sa_surf_mid.level.values, marker='o')
[<matplotlib.lines.Line2D at 0xffff51f9da90>]
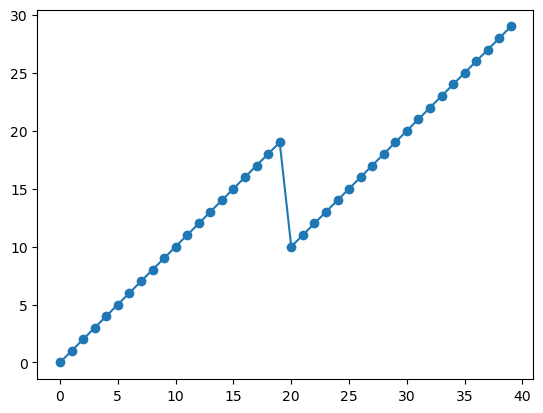
We can also concat
data along a new dimension, e.g.
sa_concat_new = xr.concat([sa_surf, sa_mid], dim='newdim')
sa_concat_new
<xarray.DataArray 'salinity' (newdim: 2, level: 30, date: 75)> Size: 36kB array([[[35.6389389 , 35.51495743, 35.57297134, ..., 35.82093811, 35.77793884, 35.66891098], [35.63393784, 35.5219574 , 35.57397079, ..., 35.81093216, 35.58389664, 35.66791153], [35.6819458 , 35.52595901, 35.57297134, ..., 35.79592896, 35.66290665, 35.66591263], ..., [ nan, nan, nan, ..., nan, nan, nan], [ nan, nan, nan, ..., nan, nan, nan], [ nan, nan, nan, ..., nan, nan, nan]], [[ nan, nan, nan, ..., nan, nan, nan], [ nan, nan, nan, ..., nan, nan, nan], [ nan, nan, nan, ..., nan, nan, nan], ..., [35.78895187, 35.7829895 , 35.85100555, ..., 35.84291458, 35.81891251, 35.7779007 ], [35.76794815, 35.75598526, 35.84500504, ..., 35.84891891, 35.83391571, 35.76390076], [35.75194168, 35.71097565, 35.83100128, ..., 35.80690765, 35.85292053, 35.75489807]]]) Coordinates: * level (level) int64 240B 0 1 2 3 4 5 6 7 8 ... 21 22 23 24 25 26 27 28 29 * date (date) datetime64[ns] 600B 2012-07-13T22:33:06.019200 ... 2014-0... lon (date) float64 600B -39.13 -37.28 -36.9 ... -33.83 -34.11 -34.38 lat (date) float64 600B 47.19 46.72 46.45 46.23 ... 42.6 42.46 42.38 Dimensions without coordinates: newdim
Note that the data were aligned using an outer join along the non-concat dimensions.
We can merge both DataArrays
and Datasets
:
xr.merge([argo.salinity, argo.temperature])
<xarray.Dataset> Size: 96kB Dimensions: (level: 78, date: 75) Coordinates: * level (level) int64 624B 0 1 2 3 4 5 6 7 ... 70 71 72 73 74 75 76 77 * date (date) datetime64[ns] 600B 2012-07-13T22:33:06.019200 ... 20... lon (date) float64 600B -39.13 -37.28 -36.9 ... -34.11 -34.38 lat (date) float64 600B 47.19 46.72 46.45 ... 42.6 42.46 42.38 Data variables: salinity (level, date) float64 47kB 35.64 35.51 35.57 ... nan 34.94 nan temperature (level, date) float64 47kB 18.97 18.44 19.1 ... nan 3.714 nan
If the data are not aligned, they will be aligned before merge.
We can specify the join options in merge
.
xr.merge([
argo.salinity.sel(level=slice(0, 30)),
argo.temperature.sel(level=slice(30, None))
])
<xarray.Dataset> Size: 96kB Dimensions: (level: 78, date: 75) Coordinates: * level (level) int64 624B 0 1 2 3 4 5 6 7 ... 70 71 72 73 74 75 76 77 * date (date) datetime64[ns] 600B 2012-07-13T22:33:06.019200 ... 20... lon (date) float64 600B -39.13 -37.28 -36.9 ... -34.11 -34.38 lat (date) float64 600B 47.19 46.72 46.45 ... 42.6 42.46 42.38 Data variables: salinity (level, date) float64 47kB 35.64 35.51 35.57 ... nan nan nan temperature (level, date) float64 47kB nan nan nan nan ... nan 3.714 nan
xr.merge([
argo.salinity.sel(level=slice(0, 30)),
argo.temperature.sel(level=slice(30, None))
], join='left')
<xarray.Dataset> Size: 39kB Dimensions: (level: 31, date: 75) Coordinates: * level (level) int64 248B 0 1 2 3 4 5 6 7 ... 23 24 25 26 27 28 29 30 * date (date) datetime64[ns] 600B 2012-07-13T22:33:06.019200 ... 20... lon (date) float64 600B -39.13 -37.28 -36.9 ... -34.11 -34.38 lat (date) float64 600B 47.19 46.72 46.45 ... 42.6 42.46 42.38 Data variables: salinity (level, date) float64 19kB 35.64 35.51 35.57 ... 35.83 35.76 temperature (level, date) float64 19kB nan nan nan ... 13.59 13.74 13.31
20.5. Reductions#
Similar to numpy
, we can reduce xarray
DataArray
s along any number of axes:
argo.temperature.mean(axis=0)
<xarray.DataArray 'temperature' (date: 75)> Size: 600B array([10.88915385, 10.7282564 , 10.9336282 , 10.75679484, 10.38166666, 10.08619236, 10.58194804, 10.50066671, 10.56841555, 10.53705122, 10.81131168, 11.01932052, 11.39205196, 11.40823073, 11.3642208 , 11.35821797, 11.39444157, 11.10514098, 11.02870125, 10.80894868, 10.93076625, 11.01069231, 11.88195654, 10.57373078, 10.66359736, 10.56573237, 11.08854546, 10.87921792, 11.21384416, 11.24991028, 11.29168825, 11.06203848, 11.32829864, 11.20401279, 11.25300001, 11.32106403, 11.40112986, 6.07053117, 11.7748052 , 11.7466795 , 12.03732056, 11.92653251, 12.08844156, 12.20543591, 12.23402598, 12.03365387, 11.9919221 , 11.92087012, 11.84273071, 11.79711684, 11.7895325 , 11.55385894, 11.19083561, 11.266282 , 11.0611948 , 11.0307179 , 11.06566232, 10.79799995, 10.787 , 10.41173077, 10.44170127, 10.32649998, 10.38242857, 10.88080769, 10.86177921, 10.98787178, 10.93602596, 10.73039743, 11.09251948, 10.93983334, 10.65942862, 11.01814097, 11.21918184, 11.19080765, 11.13364934]) Coordinates: * date (date) datetime64[ns] 600B 2012-07-13T22:33:06.019200 ... 2014-0... lon (date) float64 600B -39.13 -37.28 -36.9 ... -33.83 -34.11 -34.38 lat (date) float64 600B 47.19 46.72 46.45 46.23 ... 42.6 42.46 42.38
argo.temperature.mean(axis=1)
<xarray.DataArray 'temperature' (level: 78)> Size: 624B array([17.60172602, 17.57223609, 17.5145833 , 17.42326395, 17.24943838, 17.03730134, 16.76787661, 16.44609588, 16.17439195, 16.04501356, 15.65827023, 15.4607296 , 15.26114862, 15.12489191, 14.99133783, 14.90160808, 14.81990544, 14.74535139, 14.66822971, 14.585027 , 14.49732434, 14.41904053, 14.35412163, 14.27102702, 14.19081082, 14.11487838, 14.04347293, 13.98067566, 13.90994595, 13.83274319, 13.76139196, 13.69836479, 13.62335132, 13.54185131, 13.46647295, 13.39395946, 13.32541891, 13.25205403, 13.18131082, 13.10233782, 12.89268916, 12.67795943, 12.4649189 , 12.2178513 , 11.98270268, 11.1281081 , 10.80430666, 10.49702667, 10.1749066 , 9.83453334, 9.48625332, 9.19793334, 8.66010666, 8.12324001, 7.60221333, 7.15289333, 6.74250667, 6.39543999, 6.04598667, 5.74538665, 5.48913333, 5.26604001, 5.08768 , 4.93479998, 4.77769334, 4.65368 , 4.54237334, 4.44274664, 4.35933333, 4.2653784 , 4.17290539, 4.08902703, 3.99864865, 3.92163514, 3.85617567, 3.78916217, 3.72950001, 3.66207691]) Coordinates: * level (level) int64 624B 0 1 2 3 4 5 6 7 8 ... 69 70 71 72 73 74 75 76 77
However, instead of performing reductions on axes (as in numpy
), we can perform them on dimensions. This turns out to be a huge convenience:
argo_mean = argo.mean(dim='date')
argo_mean
<xarray.Dataset> Size: 2kB Dimensions: (level: 78) Coordinates: * level (level) int64 624B 0 1 2 3 4 5 6 7 ... 70 71 72 73 74 75 76 77 Data variables: salinity (level) float64 624B 35.91 35.9 35.9 35.9 ... 34.94 34.94 34.93 temperature (level) float64 624B 17.6 17.57 17.51 ... 3.789 3.73 3.662 pressure (level) float64 624B 6.435 10.57 15.54 ... 1.95e+03 1.999e+03
argo_mean.salinity.plot(y='level', yincrease=False)
[<matplotlib.lines.Line2D at 0xffff51e3d6d0>]
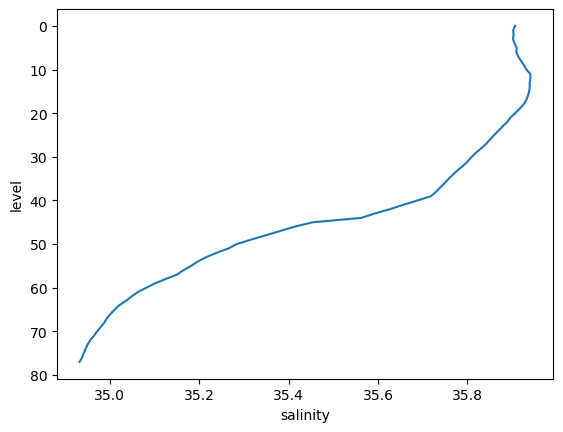
argo_std = argo.std(dim='date')
argo_std.salinity.plot(y='level', yincrease=False)
[<matplotlib.lines.Line2D at 0xffff51ecc410>]
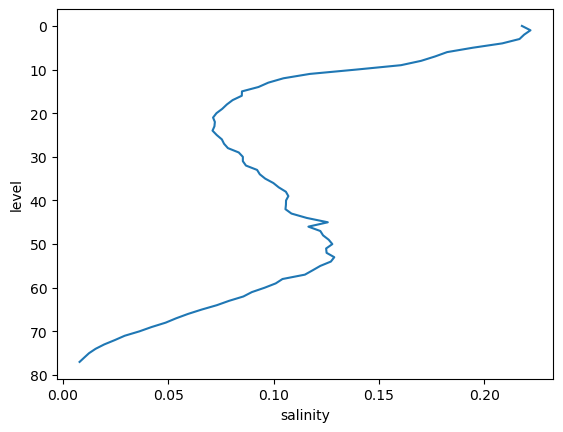
20.5.1. Weighted Reductions#
Sometimes we want to perform a reduction (e.g. a mean) where we assign different weight factors to each point in the array.
xarray
supports this via weighted array reductions.
As a toy example, imagine we want to weight values in the upper ocean more than the lower ocean. We could imagine creating a weight array exponentially proportional to pressure as following:
mean_pressure = argo.pressure.mean(dim='date')
p0 = 250
weights = np.exp(-mean_pressure / p0)
weights.plot()
[<matplotlib.lines.Line2D at 0xffff51d2f110>]
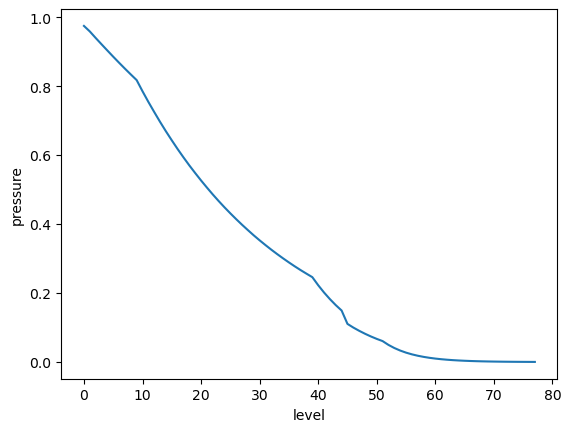
The weighted mean over the level
dimensions is calculated as follows:
temp_weighted_mean = argo.temperature.weighted(weights).mean(dim='level')
Comparing to the unweighted mean, we see the difference:
temp_weighted_mean.plot(label='weighted')
argo.temperature.mean(dim='level').plot(label='unweighted')
plt.legend()
<matplotlib.legend.Legend at 0xffff58e47620>
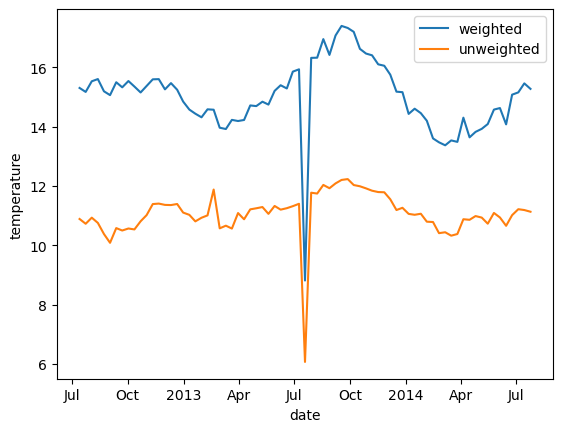